Self-Improving Autonomous AI: Revolutionizing R&D in Material Science, Drug Discovery, and Climate Solutions
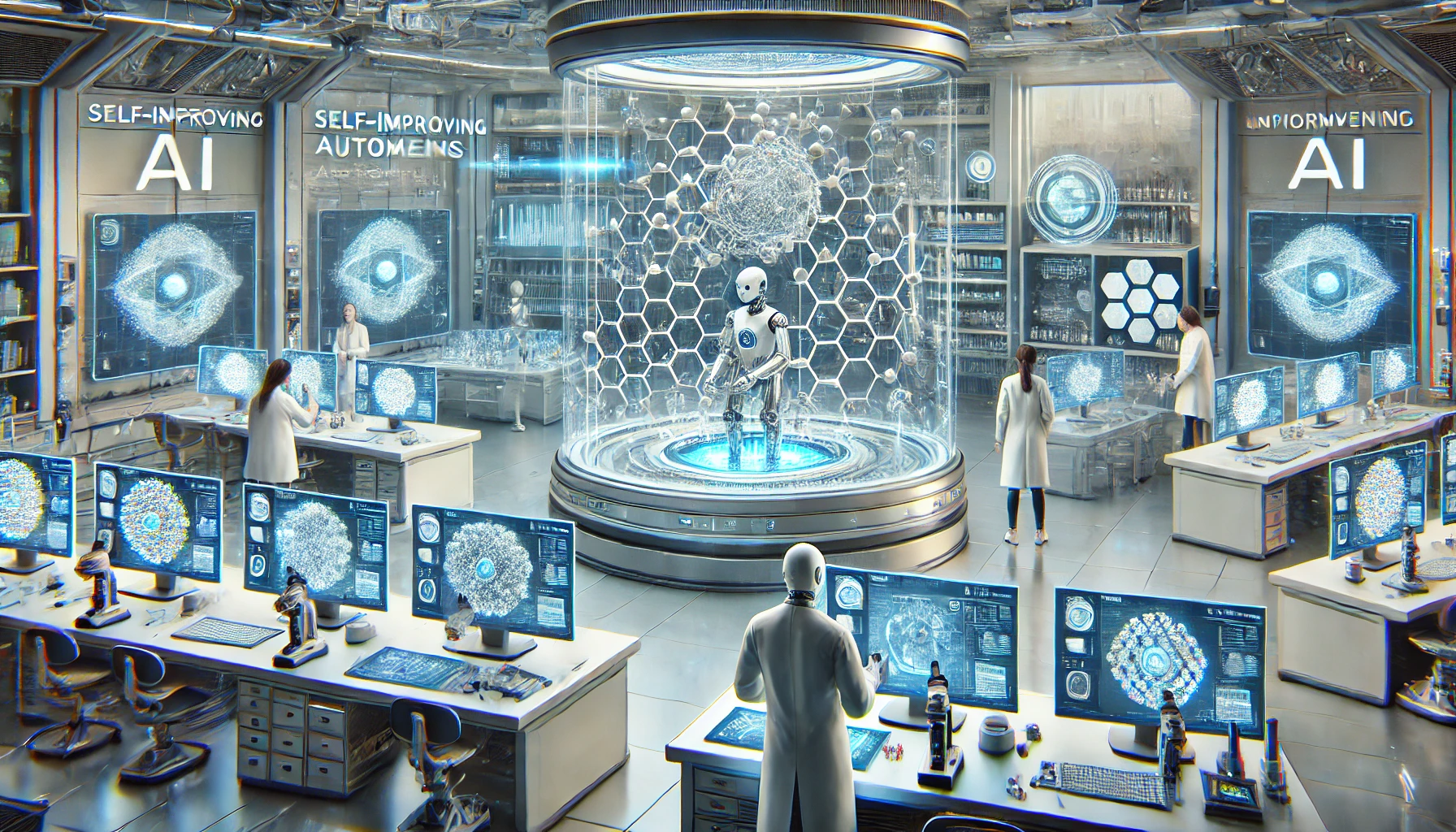
Introduction: Why Autonomous AI is the Future of R&D
The rapid integration of AI into research and development is no longer just a vision; it's a reality reshaping fields like material science, drug discovery, and climate solutions. At the forefront of this movement are self-improving AI agents—algorithms designed to learn from data and continuously enhance their own performance without constant human intervention. These systems are not only accelerating discoveries but also changing the traditional R&D landscape, promising unprecedented efficiency and breakthrough potential. In this article, we will examine the pivotal role autonomous AI plays in modern research, delve into its origins, current challenges, and explore the promising future it holds for scientific innovation.
The Evolution of Self-Improving AI: From Rule-Based Systems to Autonomous Agents
The history of self-improving AI is rooted in early computational efforts to simulate human learning. Early AI systems operated on fixed rules, requiring manual reprogramming for any adjustment. With the advent of machine learning, particularly reinforcement learning, AI systems started to adopt self-learning behaviors. These algorithms could now experiment, learn from their actions, and autonomously optimize outcomes—a feature with immense implications for R&D. An example can be seen in early machine learning models that mimicked neural networks, setting the stage for today's self-improving agents that support complex R&D tasks. This section dives into historical milestones, illustrating how foundational AI principles evolved into today's autonomous, self-learning systems.
Modern Challenges: Ethical, Technical, and Practical Hurdles in Self-Improving AI
While the benefits are clear, the application of self-improving AI in R&D brings significant challenges. Ethical concerns arise in sectors like medicine, where errors can have severe consequences. For instance, autonomous AI in drug discovery must navigate ethical frameworks to ensure patient safety. On a technical level, the substantial computational resources required can make these agents prohibitively expensive, especially for small research facilities. Statistics reveal that training a large self-improving AI model can require millions of dollars, a cost that’s out of reach for most institutions. Practical hurdles also include biases in training data that can skew results, necessitating rigorous validation to maintain research integrity. This section examines these challenges in detail, supported by examples and data from recent studies.
Real-World Applications: Autonomous AI in Action Across Industries
Across multiple fields, self-improving AI is already making an impact. In drug discovery, companies like Atomwise and BenevolentAI leverage autonomous agents to simulate molecular interactions, allowing researchers to discover new drugs more rapidly. Climate modeling also benefits as AI agents predict complex environmental patterns that help in creating effective climate solutions. Material science has seen breakthroughs where autonomous agents simulate atomic interactions, reducing the trial-and-error process in material creation. These examples, backed by current data, illustrate the diverse applications of autonomous AI, highlighting both its transformative potential and the unique industry-specific challenges it faces.
Best Practices for Implementing Self-Improving AI in R&D
Implementing self-improving AI in R&D requires a strategic approach to maximize benefits and mitigate risks. Collaborative, cross-disciplinary teams ensure that AI models are used responsibly and effectively. Regular validation cycles, where humans assess the AI’s findings, maintain research standards while minimizing risks. For instance, material science teams at top research universities have integrated these agents in iterative lab testing to ensure accuracy. Practices such as clear documentation, transparency in decision-making processes, and adherence to ethical standards are becoming the gold standard for deploying self-improving AI in critical research settings. These strategies are essential to maximize the accuracy, safety, and reliability of autonomous AI in R&D applications.
Emerging Trends and Future Implications of Self-Improving AI in Science
The future of self-improving AI holds significant implications for global science and technology. Emerging trends suggest that as AI algorithms become more sophisticated, they could significantly reduce timeframes for critical discoveries. For example, in pharmaceuticals, AI agents may reduce drug discovery time from years to months, reshaping how the industry operates. Climate research, too, could see advancements as these agents assist in real-time climate modeling, supporting more effective global response strategies. However, these advancements come with the need for careful regulation and governance to ensure responsible use. This section explores how self-improving AI may shape future R&D, the societal impact of accelerated discoveries, and the regulatory frameworks required to harness its full potential safely.
Conclusion: The Transformative Potential of Autonomous AI in R&D
Autonomous, self-improving AI agents are not just tools—they represent a new era in R&D, enabling researchers to tackle previously insurmountable challenges. As these technologies continue to evolve, they promise to redefine traditional research timelines and deliver faster, more accurate discoveries. However, realizing their full potential will require balancing innovation with ethical responsibility. As we look to the future, the question remains: how will society adapt to this new wave of autonomous science? Join the conversation on Reddit or Twitter to share your thoughts on the future of self-improving AI in R&D.