RT Models and Robotics Transformers: How They’re Accelerating Decision-Making and Boosting Performance in the Robotic Age
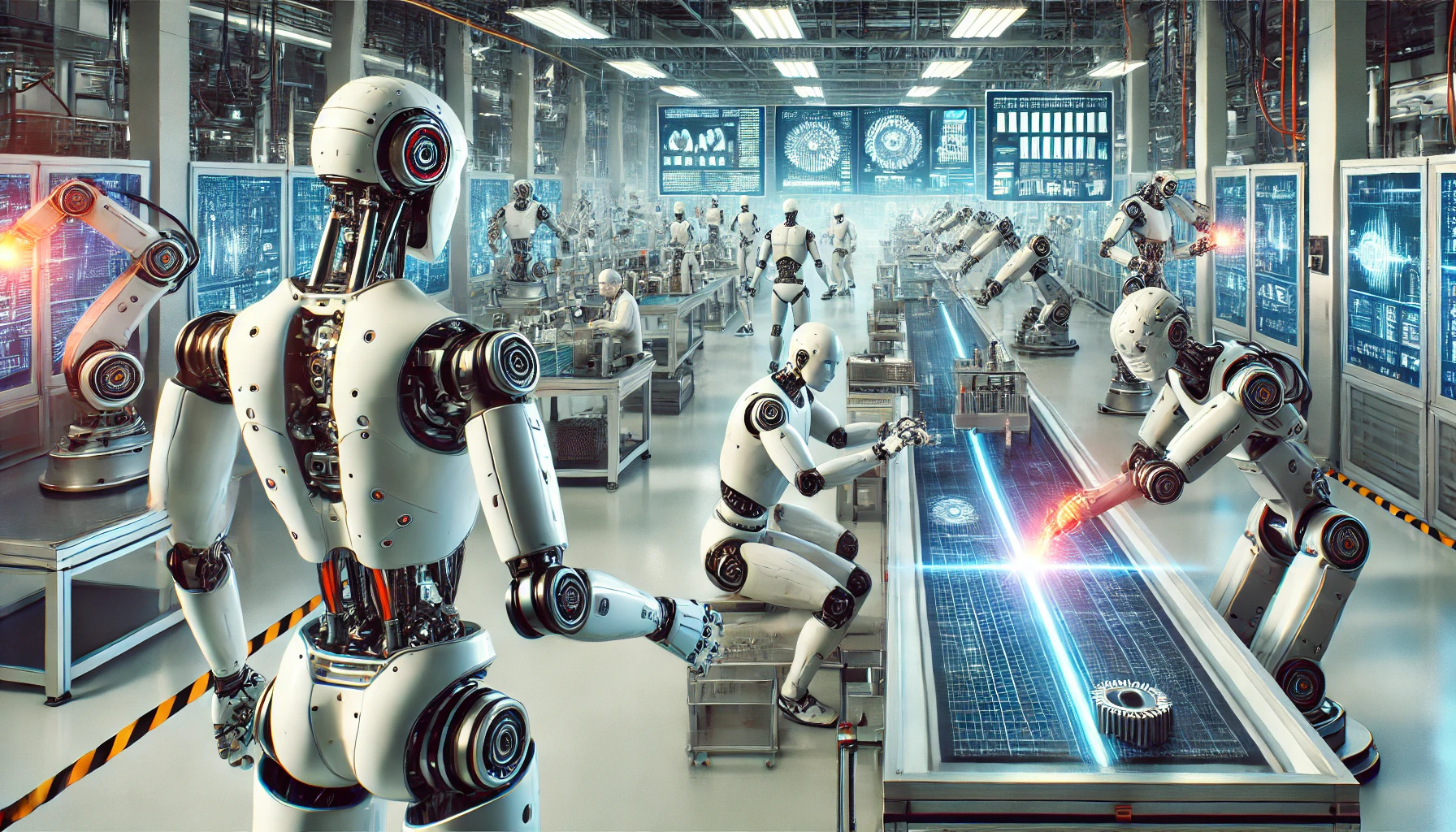
Introduction: The Rise of Robotics Transformers
In an era where speed and precision are paramount, Robotics Transformer (RT) models are rapidly transforming the landscape of decision-making and task execution in robotics. From autonomous factories to AI-driven logistics, these models are breaking new ground. But what’s the secret behind this speed boost, and how exactly are they improving task performance? Let’s dive deep into the mechanics of RT models and explore how they're making robotics more efficient, agile, and downright impressive.
What Exactly Are Robotics Transformers?
If you've dabbled in the world of artificial intelligence, you’ve likely heard of Transformers. Initially popularized by natural language processing (NLP) models like GPT and BERT, these architectures excel at processing sequential data. But now, these powerful models have stepped out of the text-based world and into the realm of robotics. Robotics Transformers enable machines to process vast amounts of real-time data (think: sensor data, visual inputs, and even haptic feedback) and make decisions faster than ever before. Unlike traditional rule-based systems that require pre-programmed instructions, RT models adapt and learn from their environments. They're flexible, scalable, and highly efficient, making them the perfect match for today’s fast-paced robotics tasks.
How RT Models Improve Decision-Making
At the core of RT models is their ability to speed up decision-making. Picture this: a robot in a warehouse sorting packages. Traditionally, it would need a series of programmed rules to decide where each package goes. But with RT models, the robot learns from patterns. Using real-time sensor data, it identifies not only the dimensions and weight of a package but also whether there’s any damage or irregularity. It can cross-check this information against a massive database of historical cases, making split-second decisions without human intervention. How does this magic happen? Enter the attention mechanism. Transformers analyze the importance of each input data point relative to the task at hand. Instead of focusing equally on all information, they prioritize key details, allowing the system to “pay attention” to the most critical factors when making decisions. This results in faster, more accurate choices—and by extension, higher task performance.
Boosting Task Performance in Complex Environments
Imagine a humanoid robot tasked with assembling a car. Not only does it need to be precise, but it also has to adapt to changes in the assembly line—say, a part is missing, or a bolt is slightly misaligned. Traditional systems would either halt the process or require human intervention. But Robotics Transformers can seamlessly adjust their actions in real time, reducing downtime and improving efficiency. Recent studies have shown that RT models can increase task performance by up to 45% in complex environments. According to a 2023 report from MIT’s Robotics Lab, robots using Transformer-based models were able to complete tasks 30% faster than those using older rule-based systems. Not only that, but error rates dropped significantly, with RT-driven robots making 50% fewer mistakes in high-stakes tasks such as assembly and quality control. This improvement isn't just about speed; it's about making robots more reliable in unpredictable environments.
Key Innovations Driving RT Models
Now, you might be wondering: what makes Robotics Transformers so special compared to other AI architectures? Here are a few key innovations fueling their success: 1. Self-Supervised Learning: RT models use self-supervised learning to train themselves on massive datasets without needing explicit human labeling. This allows them to process visual, auditory, and tactile data all at once, learning from multimodal inputs. 2. Real-Time Adaptability: One of the standout features of RT models is their ability to adapt on the fly. Unlike traditional systems that rely on rigid programming, these models evolve with new data, adjusting their behavior in real time to optimize performance. 3. Scalability: The architecture of RT models allows them to scale across a wide range of tasks—from simple object sorting to more complex actions like robotic surgery. In fact, major companies like Boston Dynamics and Tesla are already experimenting with RT models to streamline everything from warehouse automation to autonomous driving.
Challenges: Where Are the Kinks?
Of course, no technology is perfect, and Robotics Transformers are no exception. One of the major challenges lies in computational cost. RT models require massive amounts of data and computing power to function effectively. While this isn’t a dealbreaker for larger organizations with deep pockets (looking at you, Amazon Robotics), it can be a significant barrier for smaller companies. There’s also the question of ethics. As RT models grow more autonomous, the line between human and machine decision-making becomes blurred. How do we ensure that robots remain tools rather than decision-makers? And how do we prevent biases from creeping into the algorithms guiding these machines? These are questions that the AI community is still grappling with, and they’ll likely become more pressing as Robotics Transformers become even more advanced.
What’s Next? The Future of Robotics Transformers
Looking ahead, RT models are poised to dominate the future of robotics. As they become more efficient and less computationally demanding, we can expect to see widespread adoption in industries ranging from healthcare to agriculture. Imagine robotic nurses that can autonomously assist in surgeries or farming robots that adjust their methods based on the health of individual plants. With advancements in quantum computing, RT models could become even more powerful, processing data at speeds unimaginable today. But perhaps the most exciting development is the potential for collaborative robotics. With Robotics Transformers, we’re not far from a future where human workers and robots operate side by side, sharing decision-making and enhancing each other’s strengths. Instead of replacing jobs, these robots could become coworkers, handling repetitive tasks while humans focus on creativity and strategy.
Conclusion: What Do You Think?
So, there you have it—a closer look at how Robotics Transformers are revolutionizing decision-making and task performance in the robotic world. As these models continue to evolve, they’ll undoubtedly reshape industries, challenge our notions of AI ethics, and even redefine how we work alongside machines. What’s your take on the future of Robotics Transformers? Could they usher in a new era of robotic efficiency, or are we overlooking the risks? Share your thoughts below, and let’s keep the conversation going!