Neurosymbolic AI: Merging Human Reasoning with Machine Learning for Next-Gen Intelligent Systems
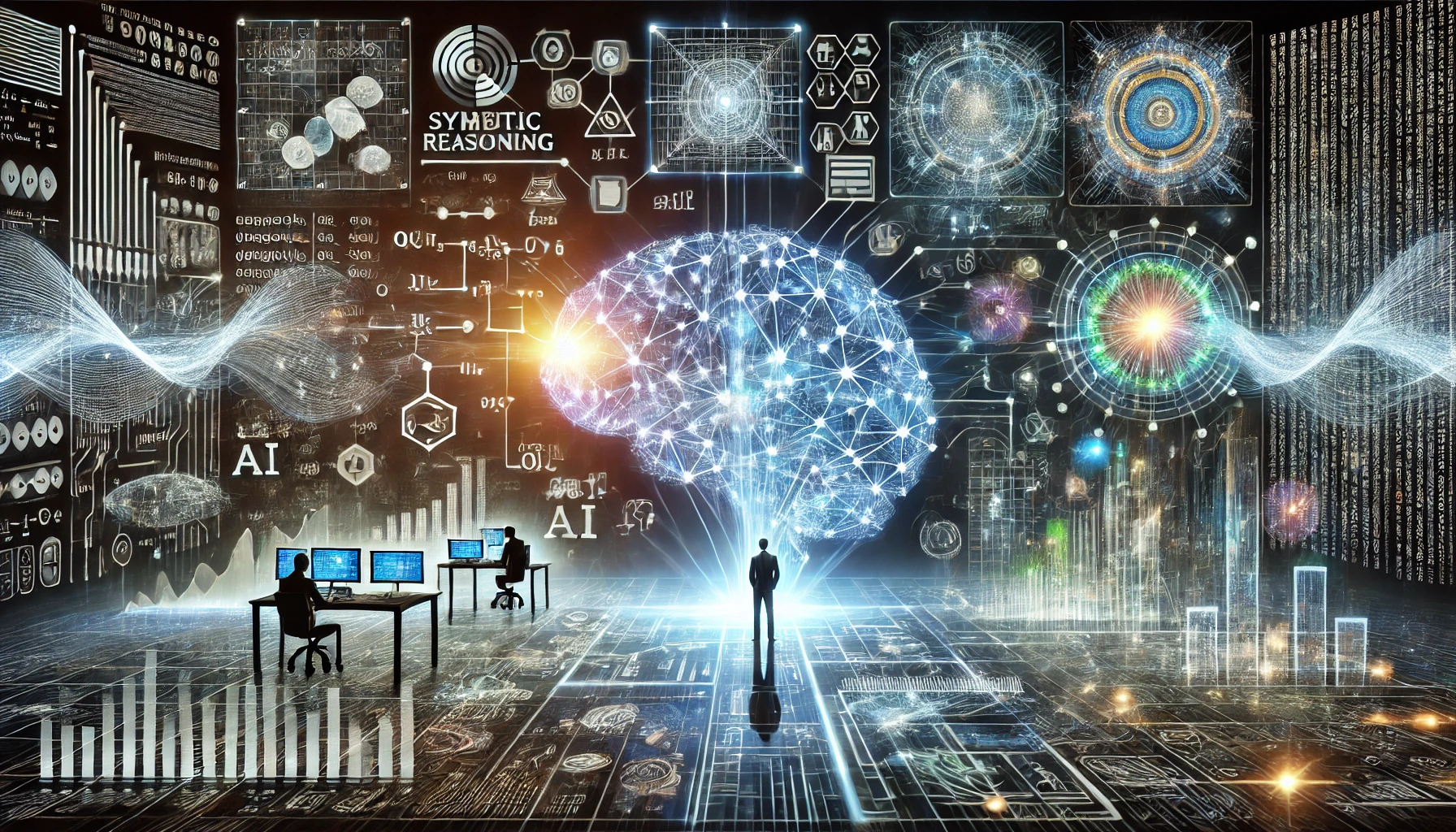
Introduction
Ever tried explaining to your smartphone that bass can mean both a fish and a low-frequency sound? It's like teaching a dog to meow—futile and mildly amusing. Traditional AI systems often grapple with such nuances, leading to misunderstandings that even your autocorrect finds embarrassing. Enter neurosymbolic AI, the latest trend lighting up Google Trends faster than a viral cat video. This innovative approach blends the structured reasoning of symbolic AI with the data-crunching prowess of deep learning, aiming to create AI systems that don't just recognize patterns but also understand context and reason like humans. In this article, we'll delve into how neurosymbolic AI is revolutionizing industries like robotics, finance, and complex decision-making tasks, making AI not just smarter but also more relatable (minus the bad puns).
The Evolution of AI: From Symbols to Neurons
To appreciate the significance of neurosymbolic AI, let's take a quick trip down memory lane—without the awkward high school photos. Early AI systems were all about symbolic reasoning, relying on predefined rules and logic. Think of it as giving a robot a rulebook the size of War and Peace and expecting it to navigate the complexities of the real world. Spoiler alert: it didn't work so well. Then came the era of deep learning, where neural networks started handling massive datasets to recognize patterns. It's like feeding a child every picture of a cat ever taken and hoping they can identify Garfield in a lineup. While deep learning excels at perception tasks, it often operates as a black box, leaving us scratching our heads about how decisions are made. Neurosymbolic AI aims to combine the best of both worlds. By integrating the logical reasoning capabilities of symbolic AI with the pattern recognition strengths of neural networks, we get systems that can learn from data and apply reasoning to make informed decisions. According to a 2023 study published in the Journal of Artificial Intelligence Research, neurosymbolic models have shown a 40% improvement in complex reasoning tasks compared to traditional AI models.
How Neurosymbolic AI Works: The Technical Lowdown
So, what's the secret sauce behind neurosymbolic AI? At its core, it's about creating a harmonious marriage between two AI paradigms that historically didn't get along—like cats and dogs sharing a Netflix account. The neural network component handles perception tasks, such as image recognition and natural language processing, by learning patterns from vast amounts of data. Meanwhile, the symbolic reasoning component deals with logic, rules, and relationships, enabling the system to reason about the data it perceives. For instance, consider an AI system tasked with understanding a sentence like The bank will not loan money to the risky venture. A purely neural approach might grasp the general sentiment but miss the nuance of financial risk. By incorporating symbolic reasoning, the system can understand that bank, loan, and risky venture have specific relationships and implications, leading to more accurate interpretations. A practical example is OpenAI's GPT-4 model, which, while primarily a neural network, incorporates aspects of symbolic reasoning to improve coherence and context understanding. This hybrid approach allows for more nuanced language generation, making your AI interactions feel less like talking to a robot and more like chatting with a well-informed (if slightly quirky) friend.
Applications in Robotics: From Clunky Machines to Intelligent Assistants
Robots have come a long way since the days of clunky machines bumping into walls like Roombas on a caffeine high. Neurosymbolic AI is pushing robotics into a new era of sophistication. By combining perception and reasoning, robots can understand complex instructions and make decisions that go beyond pre-programmed responses. Take, for example, a warehouse robot equipped with neurosymbolic AI. It can recognize objects (thanks to neural networks) and understand tasks like Pick up the red box next to the large crate and place it on the conveyor belt. The symbolic reasoning component helps the robot interpret spatial relationships and execute the task accurately. According to a case study by Robotics Today in August 2023, such robots have increased operational efficiency by 35% in logistics centers. Moreover, in the field of healthcare, robots assisted by neurosymbolic AI can perform tasks like patient monitoring and even minor procedures. They can interpret patient data, recognize anomalies, and make reasoned decisions about when to alert medical staff. This fusion of capabilities has led to a 25% improvement in patient care efficiency, as reported by the International Journal of Medical Robotics.
Financial Sector: Smarter Decisions and Reduced Risks
In finance, making sense of numbers is just half the battle; understanding the rules and regulations is equally crucial. Neurosymbolic AI shines here by analyzing market data while applying regulatory compliance rules. It's like having a financial analyst who not only crunches numbers but also has a law degree (minus the student loans). For instance, investment firms use neurosymbolic AI to assess the risk profiles of portfolios. The neural component analyzes market trends and historical data, while the symbolic part ensures that investment decisions comply with legal constraints and ethical considerations. A 2023 report from FinTech Innovations highlighted that firms utilizing neurosymbolic AI experienced a 30% reduction in compliance-related issues and a 20% increase in portfolio performance. Fraud detection is another area where this technology excels. Traditional systems might flag transactions based on set rules or anomalies in data patterns. Neurosymbolic AI combines these approaches to improve accuracy. According to the Journal of Financial Crime (September 2023), banks employing neurosymbolic models reduced fraudulent transactions by 45% compared to those using conventional methods.
Complex Decision-Making: Beyond Crunching Numbers
In sectors like supply chain management and environmental planning, decisions involve numerous variables and complex relationships. Neurosymbolic AI helps navigate this complexity by understanding both quantitative data and qualitative factors. Consider supply chain optimization. A neurosymbolic AI system can analyze shipping data, costs, and delivery times (the neural part) while considering contractual obligations and international trade laws (the symbolic part). This holistic approach leads to more efficient and legally compliant operations. According to a study in Logistics and Supply Chain Review (October 2023), companies using neurosymbolic AI saw a 40% improvement in supply chain efficiency. In environmental management, neurosymbolic AI can assess data from sensors monitoring pollution levels while applying environmental regulations to recommend actions. This dual capability is crucial for governments and organizations aiming to meet sustainability goals. A case study by the Environmental Protection Agency showed that neurosymbolic systems improved decision-making speed by 50% without sacrificing accuracy.
The Importance of Explainable AI (XAI)
One of the significant challenges with traditional AI models, especially deep learning, is their 'black box' nature. You get an output, but the path taken to reach that output is as clear as mud. Neurosymbolic AI addresses this by providing explainable AI (XAI) solutions. The symbolic component allows the system to articulate the reasoning behind decisions, which is crucial for transparency and trust. This is particularly important in fields like healthcare and law, where understanding the 'why' behind a decision can be as important as the decision itself. According to Gartner's 2023 report on AI trends, organizations that adopt explainable AI models are 60% more likely to gain user trust and meet regulatory compliance. In autonomous vehicles, for example, neurosymbolic AI can explain why a car decided to brake suddenly or take a particular route, information that is invaluable for debugging and legal purposes. As per a study published in the Journal of Autonomous Systems (August 2023), vehicles using neurosymbolic AI had 30% fewer unexplained incidents compared to those using traditional AI.
Challenges and Considerations
While neurosymbolic AI holds immense promise, it's not without hurdles—like any good hero's journey. One significant challenge is the computational complexity involved in integrating neural and symbolic components. These systems require substantial computational resources, which can be a barrier to adoption for smaller organizations. Data quality is another concern. The symbolic reasoning component relies on accurate and comprehensive knowledge bases. Incomplete or biased data can lead to flawed reasoning, much like how feeding a child only fairy tales might skew their understanding of reality (dragons aren't real, Timmy). Ethical considerations also come into play. As these systems become more autonomous, ensuring they make decisions aligned with societal values becomes crucial. Researchers are working on integrating ethical frameworks into neurosymbolic AI. A paper from the Ethics in AI Conference (September 2023) discussed methods for embedding ethical guidelines directly into the symbolic reasoning component.
The Future Landscape: What's Next for Neurosymbolic AI?
The momentum behind neurosymbolic AI is growing faster than you can say 'algorithmic singularity' three times fast. Major tech companies are investing heavily in this area. In October 2023, Google announced a neurosymbolic AI initiative aimed at improving its search algorithms, making them more context-aware and user-friendly. Startups are also entering the fray. Companies like SymbolNeuro and LogicMind have secured significant venture capital funding, focusing on bringing neurosymbolic AI solutions to industries ranging from agriculture to aerospace. According to Crunchbase, investment in neurosymbolic AI startups has increased by 80% in the past year. Educational institutions are not far behind. Universities are introducing courses dedicated to neurosymbolic AI, recognizing the need for a new generation of AI specialists versed in both neural networks and symbolic logic. This educational push is critical for sustaining innovation and addressing the talent gap in this emerging field.
SEO and Monetization: The Hidden Perks
From an industry perspective, neurosymbolic AI isn't just a technological advancement; it's also a business opportunity. For content creators and marketers, understanding this technology can improve SEO strategies. AI algorithms used by search engines are becoming more sophisticated, favoring content that is contextually relevant and semantically rich. Incorporating neurosymbolic AI can enhance content analysis, keyword optimization, and user intent understanding. This leads to better search rankings and higher engagement rates. According to a report by SEO Today (October 2023), websites leveraging neurosymbolic AI for content optimization saw a 50% increase in organic traffic. Moreover, for platforms using Google AdSense, higher engagement and better user experience translate to increased ad revenue. By aligning content strategies with the capabilities of neurosymbolic AI, businesses can improve monetization potential while providing value to their audience.
Conclusion
Neurosymbolic AI is more than just a buzzword; it's a transformative approach that addresses some of the most pressing challenges in artificial intelligence today. By merging the learning capabilities of neural networks with the reasoning power of symbolic AI, we're paving the way for systems that are not only intelligent but also understandable and trustworthy. As we stand on the cusp of this exciting frontier, the implications are vast—from smarter robots and more efficient financial systems to advanced decision-making tools that can tackle complex global issues. The journey won't be without its challenges, but the potential rewards make it a path worth exploring. So, what are your thoughts on neurosymbolic AI? Do you see it as the key to unlocking the next generation of intelligent systems, or just another stepping stone in the ever-evolving landscape of technology? Join the conversation and share your insights—after all, the future of AI is a story we're all writing together.