How Digital Twins Are Revolutionizing Personalized Medicine: The Future of Patient Care
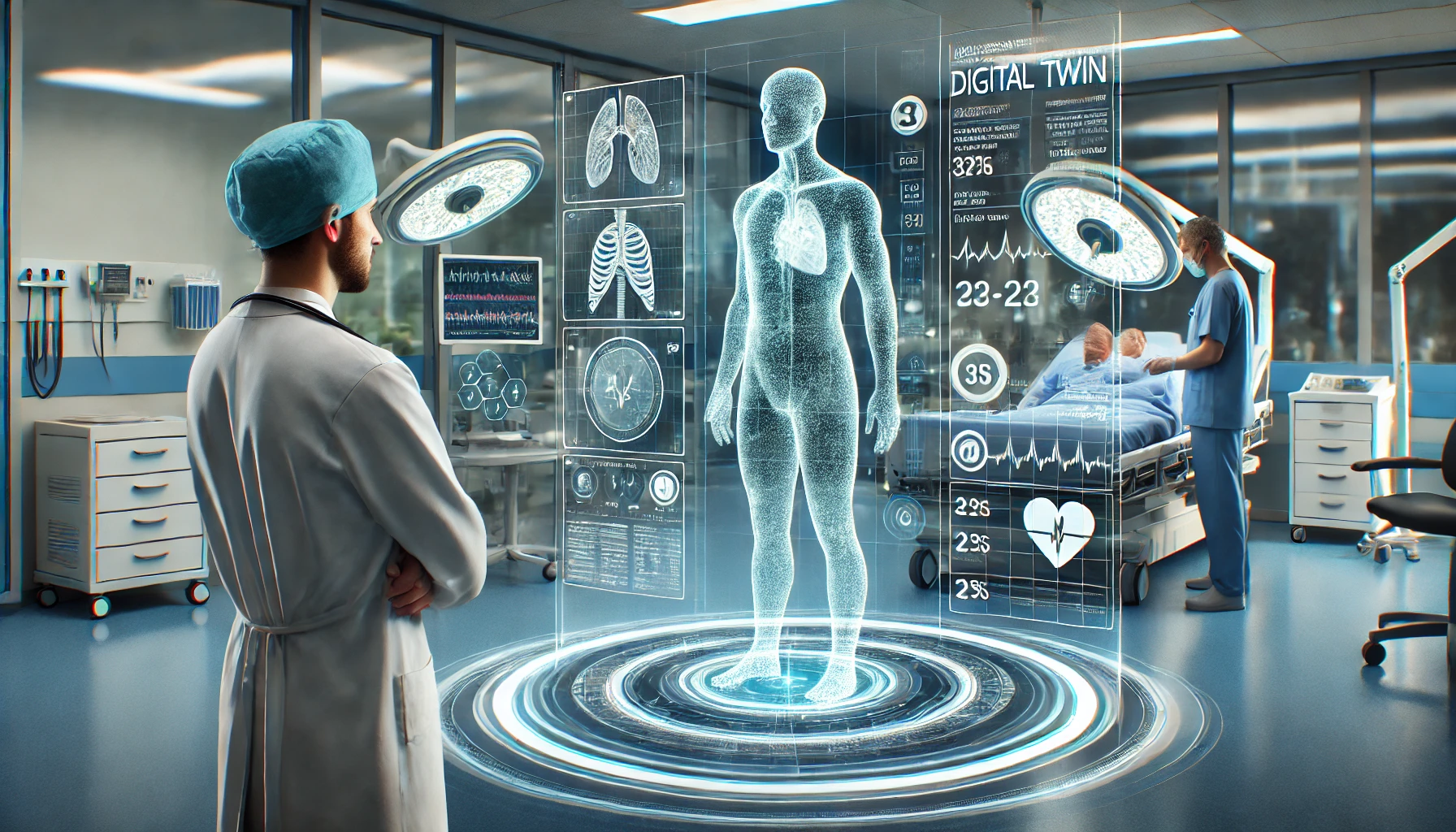
The Growing Importance of Digital Twins in Personalized Medicine
In today’s evolving healthcare landscape, digital twins have emerged as transformative tools for personalized medicine. A digital twin is a virtual replica of a patient that simulates health conditions, treatment responses, and potential disease progressions, allowing healthcare providers to tailor treatments with unprecedented precision. This trend is particularly important given the rising costs of healthcare and the shift toward preventive and patient-centered care models. By providing detailed simulations, digital twins can anticipate health issues before they arise, thus reducing hospital admissions, improving patient outcomes, and lowering overall healthcare expenses.
Origins and Evolution of Digital Twins in Healthcare
The concept of digital twins originates in the manufacturing and aerospace industries, where virtual replicas of physical assets were used to predict maintenance needs and optimize performance. In healthcare, digital twins are now applied to model patients’ unique physiology, which marks a leap from the use of simple health data records. Early developments in the field saw the integration of simulation techniques in medical education, but recent advancements in AI and data analytics have enabled healthcare providers to create dynamic, adaptable models of individual patients. Digital twins in healthcare go beyond traditional diagnostics and imaging, evolving into tools for predicting patient responses to treatments and personalizing care to suit their specific biological makeup, akin to creating a ‘virtual doppelgänger’ of each patient.
Current Challenges in Implementing Digital Twins in Healthcare
Despite their potential, digital twins in healthcare face several barriers, with data privacy and security being primary concerns. Creating and maintaining digital replicas requires vast amounts of sensitive patient data, leading to heightened risks of data breaches and regulatory concerns. Furthermore, integrating digital twins with existing hospital systems is challenging due to the outdated infrastructure still present in many facilities. Financial constraints and the high costs of implementing digital twin technology also present obstacles, especially in countries with less robust healthcare funding. Statistics show that fewer than 20% of global healthcare institutions currently have the infrastructure to support such advanced technologies, which highlights the significant gap between digital twin potential and actual application.
Real-World Examples of Digital Twin Technology in Action
Several companies are making strides in the field of digital twin technology, each bringing unique applications to personalized medicine. For instance, GE Healthcare's digital twin technology allows healthcare providers to simulate different treatment scenarios for patients with chronic conditions, which helps optimize their care plans. Siemens Healthineers has also adopted digital twin models to monitor cardiovascular health, which enables doctors to make more informed treatment decisions. In the US, digital twins have been used in cancer treatment to simulate patient-specific responses to various chemotherapy drugs, while Germany has integrated digital twin models to monitor and adjust treatments for patients with diabetes. These applications showcase the real-world impact of digital twins in healthcare, proving that, when properly implemented, digital twins can significantly improve patient outcomes and operational efficiencies.
Solutions and Best Practices for Adopting Digital Twins in Healthcare
To navigate the challenges associated with digital twin implementation, healthcare providers can adopt several best practices. Firstly, collaboration with technology firms specializing in secure data management is essential to safeguard patient information. Implementing industry standards for data security and ensuring regulatory compliance can protect against data breaches, thus making digital twin technology safer for widespread adoption. Furthermore, training healthcare personnel on the use of digital twins and integrating machine learning algorithms can improve the predictive accuracy of the models. Institutions should prioritize pilot programs that test digital twin efficacy on smaller scales before rolling them out hospital-wide. Additionally, building strong partnerships with academic institutions can further research and innovation in digital twin technology, helping to refine best practices and optimize their use in healthcare settings.
Future Implications: Emerging Trends and Long-Term Impact on Healthcare
The future of digital twins in healthcare is bright, with emerging trends pointing toward increased accessibility and functionality. As AI and machine learning become more advanced, digital twins will likely integrate seamlessly into hospital workflows, allowing for real-time patient monitoring and proactive intervention. Future applications of digital twins could extend beyond healthcare, influencing areas like biotechnology, where personalized drug development could become the norm, and even pharmacology, where drug efficacy could be tested in virtual models before patient trials. The societal implications are also profound; by enabling personalized and preventive care, digital twins could alleviate strain on healthcare systems, reduce patient recovery times, and promote overall well-being. A future where every individual has a digital twin is becoming more plausible, promising better patient outcomes, lower healthcare costs, and a shift toward proactive rather than reactive medicine.
Conclusion and Final Thoughts
In summary, digital twins are paving the way for a more proactive, individualized healthcare system. Their ability to simulate real-world patient responses holds promise for reducing medical costs, improving outcomes, and creating a healthcare experience tailored to the individual. As digital twin technology continues to evolve, it has the potential to redefine how medical professionals approach patient care and how society interacts with healthcare systems. However, the road to full adoption is paved with challenges that must be addressed through collaboration, innovation, and a commitment to data security. What are your thoughts on the potential of digital twins in transforming healthcare? Join the discussion on Reddit or Twitter!