Bias in AI: Addressing Ethical Concerns in Machine Learning Algorithms
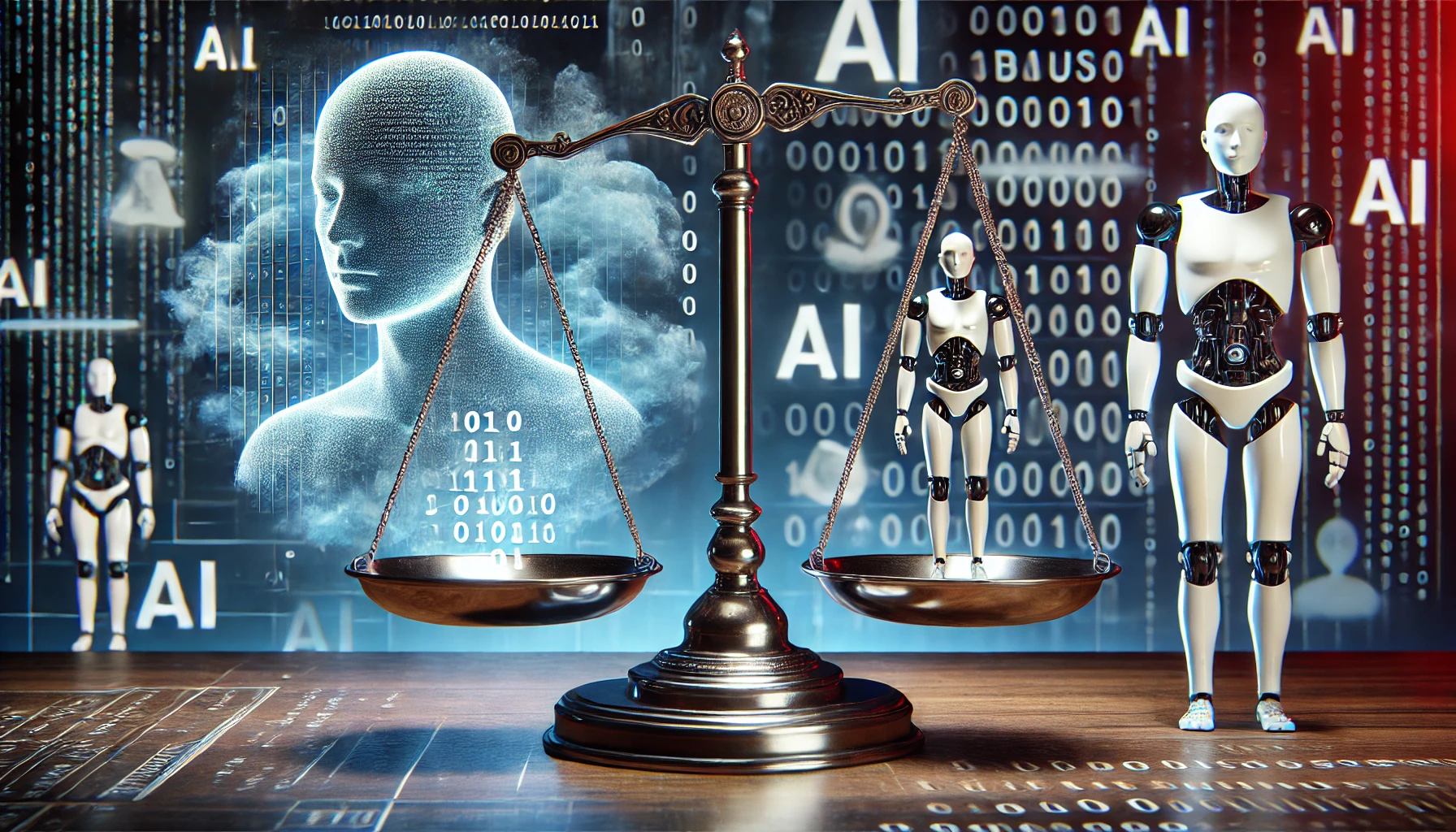
The Elephant in the Data Room: Unpacking Bias in AI
When we think of artificial intelligence, we often imagine impartial, logical systems capable of making fair decisions free from human prejudice. But, let’s face it: AI is only as good as the data it’s trained on—and here’s where things get messy. In fields like hiring and law enforcement, AI algorithms have been shown to perpetuate biases, sometimes amplifying them with alarming efficiency. For example, a recent study found that AI used in recruitment tools exhibited gender bias, favoring male candidates over female ones, even when qualifications were identical. It's a reminder that while AI might not inherently 'feel' bias, it can certainly learn and replicate it.
Algorithmic Justice: The Scale and Impact of AI Bias
The scope of AI bias extends far beyond recruitment. In law enforcement, for example, predictive policing tools have been criticized for disproportionately targeting minority communities. A 2022 report showed that in some U.S. cities, AI-driven predictive policing algorithms had a 40% higher rate of false positives for African American individuals compared to their white counterparts. Ouch. It’s like giving RoboCop a faulty radar—he’ll still catch the wrong people, but with mechanical precision. This raises serious concerns about fairness and justice in AI’s applications, especially when decisions can significantly affect lives and livelihoods.
Why Is Bias So Hard to Eliminate? Blame the Data
AI algorithms depend on historical data, and as we all know, history isn’t exactly bias-free. Whether it's hiring data that reflects decades of systemic discrimination or arrest records tainted by racial profiling, AI systems trained on such data can’t help but learn the biases ingrained in it. And since most machine learning models are designed to optimize for patterns, they tend to reinforce these inequalities unless actively mitigated. It’s like teaching a parrot to swear and then being surprised when it doesn’t hold back in front of company—bad data in, bad outcomes out.
Bias in Hiring: A Real-World Case Study
Amazon’s hiring algorithm fiasco is a prime example of AI bias in action. Back in 2018, Amazon had to scrap an AI tool designed to review resumes because it showed a bias against women. The system was trained on resumes submitted over a 10-year period, most of which came from men (hello, tech industry). As a result, the AI began to down-rank resumes that included the word 'women’s,' such as in 'women’s chess club.' Talk about a facepalm moment. This case illustrates just how easily bias can creep into AI systems, even with well-intentioned designs.
Solutions on the Horizon: Can We Teach AI to Be Fair?
There’s good news, though: the tech world isn’t sitting idly by. Techniques like algorithmic auditing, fairness constraints, and bias-mitigation frameworks are being developed to tackle these issues head-on. For instance, IBM’s AI Fairness 360 toolkit offers a suite of algorithms to help detect and reduce bias in datasets. Similarly, Google has rolled out a set of AI principles to guide the ethical use of machine learning. But let’s not kid ourselves—these solutions are not a magic bullet. Bias mitigation requires ongoing vigilance, rigorous testing, and, most importantly, diverse teams behind the algorithms. After all, you wouldn’t let one person write the whole script for humanity, right?
Beyond Data: Ethical AI Development Needs More Than Just Code
It's not just about fixing the code or tweaking algorithms. Ethical AI development also involves cultural change. We need more transparency in how AI systems are built and deployed. Companies like Microsoft and Google have created ethics boards to oversee AI projects, but the effectiveness of these initiatives remains debatable. The real challenge lies in balancing innovation with responsibility. Sure, we want our algorithms to be smart, but they also need to be socially aware. And let’s not forget: regulation is starting to catch up. The EU’s AI Act, set to be implemented in 2024, aims to establish clear guidelines for AI applications, particularly in high-risk areas like law enforcement.
What’s Next for AI Fairness? A Call for Collective Action
So, where do we go from here? First, we need to continue investing in research that addresses AI bias head-on. Academic institutions and private companies alike are pouring resources into understanding how bias creeps into machine learning systems, and how we can fix it. Second, there’s an urgent need for collaboration across industries. Governments, tech companies, and civil society must work together to create standards and regulations that ensure AI fairness. And finally, we need you—the public—to stay engaged in these conversations. After all, AI will increasingly shape our world, and it’s up to all of us to ensure it does so in a way that’s equitable and just.
Conclusion: How Can We Balance Innovation and Fairness in AI?
As we continue to push the boundaries of what AI can do, we must keep asking ourselves: how can we balance innovation with fairness? How do we ensure that the technologies we develop serve everyone, not just a privileged few? These questions are at the heart of the ethical AI debate, and there’s no one-size-fits-all answer. But the good news is that we’re making progress, albeit slowly. With the right mix of technology, policy, and public engagement, we can build AI systems that are not only powerful but also fair and just. What do you think—how should we be holding AI developers accountable for the biases their systems perpetuate?